Evidence around inequality for APPG AI
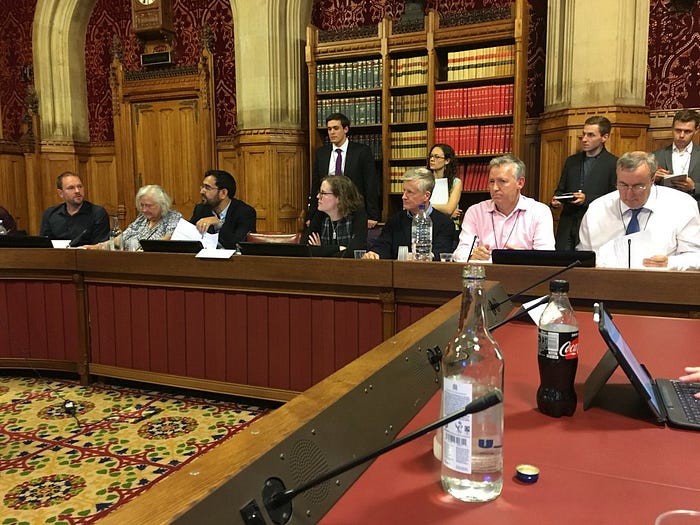
The following is my presentation, representing Doteveryone, for today’s APPG AI evidence session.
Artificial intelligence does not in and of itself reduce or create inequality. AI is a tool, and its outcomes are determined by the way we humans use it.
Currently, the biggest users and developers of AI are the organisations with access to the most expertise, data and computer hardware. These are largely private sector companies working to solve private sector problems, creating wealth for a few.
Socially important sectors, like care and education, may not benefit much from AI in the near term, if appropriate data, and investment, is not available. Furthermore, if the data that is available does not capture a sector’s breadth and human impact, AI and big data solutions more generally will not meet real human needs. These are examples of sectors or situations that are hard to measure, or where measures miss important human factors, and AI can exacerbate a metrics-driven culture which neglects human values and contact.
We must look beyond productivity and GDP, to triple bottom line (financial, environmental and societal) and other forms of measurement, to ensure we do not neglect externalities and human values in how we apply and assess the success (or otherwise) of AI.
Inequality is not just about fairness of algorithms and AI, or automation of some job types. It is about whether AI is indeed offering the benefits it promises — whether it is an effective tool. This is especially the case for under-served populations who may suffer disproportionately if promised benefits are not delivered.
We must evaluate AI critically, and avoid ‘magical thinking’ — knowing that both information and software can be wrong. Replacing humans with AI may be beneficial in some cases. But we must remember to value the human aspect and not see every task or role or decision as something that could be automated.
This is particularly important for people who need care, or whose circumstances are difficult and multifaceted. Automated decisions made here may not be sufficient or may be informed by poor quality data — a particular risk for those less able to access, evaluate and request changes to the information held about them.
Automated decisions in key areas such as justice and recruitment are already disproportionately affecting low wage earners. For example, automated job application processing is more likely to be used for high-turnover, low-skilled roles. Predictive policing is used predominantly to address street crime, rather than fraud, tax evasion and similar white-collar crimes.
As a society, we should make fuller use of the vast quantity of good-quality data that’s publicly held and collected. (ONS’s Data Science Campus is a good, but small, example of this already happening.)
Such publicly-held data, that is not open data because of personal content, could offer enormous value through AI, and this should be realised as shared public value not private wealth centralisation.
Access to this data should be granted in ways that ensure public benefit reflecting the future value which can be realised from the unlocking of insights and intelligence, and positive public outcomes.
For instance, NHS data used appropriately, and with appropriate patient involvement, could develop and advance healthcare.
There are a number of issues that need to be considered, and steps taken, for this to happen effectively and efficiently.
In the short term, there needs to be joined-up thinking, across Government and the public sector, in drawing up data and AI contracts with outside organisations.
Public bodies lack the competence and experience required to negotiate data contracts effectively, particularly with private-sector companies that have far greater experience and resources.
The drawing up of individual contracts for data deals between different public sector bodies and the same external companies — for example, the use of AI chatbots for local council services — could lead to larger costs, and a greater chance of mistakes being made.
The compliance failures in the agreement between The Royal Free Hospital and Google DeepMind exemplify many of the major issues at stake.
If Government and the public sector don’t get contract negotiation right, there is great potential for harm to privacy rights, to public trust in data sharing and use, and a great danger that valuable publicly held data assets would be handed to private companies, leading private value to be created from public assets, without appropriate recompense and increasing inequality.
Public sector bodies must take greater steps in sharing best practice around wise deal-making, learning from mistakes and successes.
Centralisation of AI contract-making should also be explored as a solution to the skills shortage in negotiation around data. The decentralisation of some services may need to be considered for this to happen, for example within regions or the NHS.
Longer term, to capture genuine public benefit from publicly held data, Government must also access its own AI expertise, and develop its talent, capacity and collaborative potential. It should not rely on corporations alone to unlock the potential.
This will require Government and the public sector to recruit and develop strong, knowledgeable, responsible AI specialists — and leaders.
The near-term costs of doing this are not insignificant. But the long-term economic benefits of building the UK’s AI capability — for the shared benefit of the population — would make the investment worthwhile.